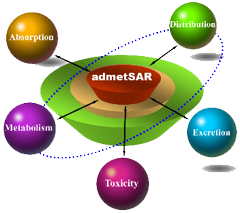
The overview of the Contrastive Learning based Multi-Task Graph Neural Network framework (CLMGraph) is shown in Figure 1. CLMGraph consists of an input module, a feature extraction module, and an ADMET predictor module.
Convert compound into a molecular graph: , where represents the node matrix and represents the edge feature matrix.
Update the node and edge information of the molecular graph using a Graph Neural Network (GNN): Optimize the GNN network by maximizing the mutual information between pairs of augmented molecular graphs using a self-supervised pre-training strategy based on contrastive learning. Use NT-Xent as the objective function to train the model: where , and are the feature vectors extracted by GNN from positive pairs and negative samples, is the batch size, is the temperature parameter, and represents cosine similarity.
Jointly train the pre-trained model on 108 ADMET prediction tasks to leverage and share relevant information across similar tasks. Optimize the ADMET prediction module using a related task loss (related task loss): Overall, the CLMGraph framework combines contrastive learning and multi-task learning to extract features from compound structures using self-supervised pre-training and joint training, leading to accurate predictions on ADMET tasks. This approach improves model performance and leverages information sharing among related tasks to accelerate learning.
Properities | Total (positive/Negative) | Training set (positive/Negative) | validation set (positive/Negative) | test set (positive/Negative) |
---|---|---|---|---|
logS | 1514 | 1080 | 275 | 159 |
logP | 13076 | 9392 | 2368 | 1316 |
pKa | 5149 | 3723 | 936 | 490 |
Acidic pKa | 2719 | 1965 | 491 | 263 |
Basic pKa | 2725 | 1954 | 514 | 257 |
Caco-2 classify | 962/749 | 679/543 | 183/130 | 100/76 |
Caco-2 | 1750 | 1255 | 316 | 179 |
HIA | 65/437 | 51/301 | 12/91 | 2/45 |
MDCK | 217/205 | 151/143 | 43/39 | 23/23 |
F50% | 854/1086 | 625/768 | 154/207 | 75/111 |
F30% | 598/1342 | 433/960 | 115/246 | 50/136 |
F20% | 445/1495 | 323/1070 | 88/273 | 34/152 |
BBB | 451/1976 | 323/1444 | 74/338 | 54/194 |
OATP1B1 inhibitor | 176/1276 | 131/914 | 36/211 | 9/151 |
OATP1B3 inhibitor | 118/1348 | 84/971 | 27/221 | 7/156 |
OATP2B1 inhibitor | 132/38 | 92/24 | 34/11 | 6/3 |
OCT1 inhibitor | 84/34 | 70/21 | 9/11 | 5/2 |
OCT2 inhibitor | 522/150 | 367/115 | 99/21 | 56/14 |
BCRP inhibitor | 483/423 | 341/306 | 87/71 | 55/46 |
BSEP inhibitor | 212/258 | 160/178 | 31/47 | 21/33 |
MATE1 inhibitor | 557/59 | 400/43 | 107/7 | 50/9 |
Pgp inhibitor | 651/1118 | 485/842 | 103/178 | 63/98 |
Pgp substrate | 797/669 | 569/490 | 149/116 | 79/63 |
PPB | 3804 | 2716 | 668 | 420 |
VDss | 1166 | 845 | 207 | 114 |
CYP1A2 inhibitor | 9037/8095 | 6564/5784 | 1568/1506 | 905/805 |
CYP3A4 inhibitor | 13790/5734 | 9932/4084 | 2491/1025 | 1367/625 |
CYP2B6 inhibitor | 306/182 | 215/135 | 58/28 | 33/19 |
CYP2C9 inhibitor | 11516/6277 | 8338/4507 | 2074/1099 | 1104/671 |
CYP2C19 inhibitor | 10087/7974 | 7301/5719 | 1799/1433 | 987/822 |
CYP2D6 inhibitor | 14264/3773 | 10269/2698 | 2577/670 | 1418/405 |
CYP1A2 substrate | 1055/457 | 746/341 | 198/71 | 111/45 |
CYP3A4 substrate | 1747/1330 | 1257/966 | 314/240 | 176/124 |
CYP2B6 substrate | 248/229 | 183/178 | 40/34 | 25/17 |
CYP2C9 substrate | 1486/511 | 1071/361 | 271/97 | 144/53 |
CYP2C19 substrate | 1038/398 | 743/284 | 200/76 | 95/38 |
CYP2D6 substrate | 1519/615 | 1104/452 | 279/98 | 136/65 |
HLM | 3871/2126 | 2800/1525 | 689/407 | 382/194 |
RLM | 2270/3294 | 1613/2361 | 419/600 | 238/333 |
UGT substrate | 537/597 | 390/441 | 95/95 | 52/61 |
CLp | 565/521 | 409/380 | 94/98 | 62/43 |
CLr | 204/280 | 152/207 | 34/43 | 18/30 |
T1/2 | 1155 | 837 | 208 | 110 |
MRT | 1165 | 844 | 209 | 112 |
Neurotoxicity | 419 | 303 | 67 | 49 |
DILI | 901/1255 | 672/903 | 145/229 | 84/123 |
hERG 1uM | 3696/797 | 2633/599 | 718/135 | 345/63 |
hERG 10uM | 1826/2667 | 1285/1947 | 360/493 | 181/227 |
hERG 30uM | 912/3581 | 630/2602 | 183/670 | 99/309 |
hERG 1-10uM | 1826/797 | 1285/599 | 360/135 | 181/63 |
hERG 10-30uM | 912/2667 | 630/1947 | 183/493 | 99/227 |
Respiratory toxicity | 997/1185 | 697/875 | 188/202 | 112/108 |
Nephrotoxicity | 350/301 | 262/215 | 62/55 | 26/31 |
Eye corrosion | 1194/855 | 849/613 | 218/141 | 127/101 |
Eye irritation | 1137/3761 | 813/2739 | 208/680 | 116/342 |
Skin corrosion | 1187/698 | 854/469 | 199/136 | 134/93 |
Skin irritation | 1187/1256 | 854/913 | 199/218 | 134/125 |
Skin sensitisation | 565/354 | 388/250 | 109/66 | 68/38 |
Acute dermal toxicity | 664/784 | 453/561 | 138/146 | 73/77 |
Ames mutagenesis | 3601/4372 | 2561/3197 | 672/762 | 368/413 |
Mouse carcinogenicity classify | 386/371 | 267/263 | 76/75 | 43/33 |
Mouse carcinogenicity | 376 | 265 | 79 | 32 |
Rat carcinogenicity classify | 474/500 | 338/349 | 92/101 | 44/50 |
Rat carcinogenicity | 498 | 345 | 105 | 48 |
Rodents carcinogenicity | 394/498 | 279/359 | 73/92 | 42/47 |
Micronucleus | 364/225 | 267/157 | 58/48 | 39/20 |
Reproductive toxicity | 917/783 | 653/553 | 175/148 | 89/82 |
Mitochondrial toxicity | 1504/1361 | 1101/981 | 253/249 | 150/131 |
Hemolytic toxicity | 496/387 | 348/271 | 97/74 | 51/42 |
Repeated dose toxicity | 674/1145 | 474/822 | 135/203 | 65/120 |
Acute oral toxicity classify | 1343/2581 | 936/1908 | 242/417 | 165/256 |
Acute oral toxicity | 13493 | 9773 | 2332 | 1388 |
FDAMDD classify | 505/428 | 380/315 | 86/72 | 39/41 |
FDAMDD | 1074 | 801 | 176 | 97 |
AR | 5745/524 | 4143/391 | 1030/87 | 572/46 |
ER | 6220/391 | 4484/293 | 1113/66 | 623/32 |
AR-LBD | 5818/542 | 4206/394 | 1037/96 | 575/52 |
ER-LBD | 5625/663 | 4047/502 | 1021/104 | 557/57 |
Aromatase | 4977/655 | 3593/473 | 887/117 | 497/65 |
AhR | 5205/707 | 3782/509 | 916/138 | 507/60 |
ARE | 4256/814 | 3030/606 | 791/145 | 435/63 |
ATAD5 | 6162/328 | 4457/254 | 1094/47 | 611/27 |
HSE | 5295/318 | 3807/238 | 954/51 | 534/29 |
p53 | 5772/404 | 4170/297 | 1038/74 | 564/33 |
PPARγ | 5949/471 | 4316/335 | 1046/95 | 587/41 |
MMP | 4214/797 | 3026/586 | 769/135 | 419/76 |
TR | 4578/331 | 3272/249 | 847/52 | 459/30 |
GR | 4946/549 | 3555/390 | 893/110 | 498/49 |
Honey bee toxicity | 462/158 | 333/117 | 83/23 | 46/18 |
Avain toxicity (Colinus virginanus) | 395/84 | 282/71 | 71/9 | 42/4 |
Avain toxicity (Anas platyrhynchos) | 416/82 | 310/62 | 65/14 | 41/6 |
Aquatic toxicity (P. subcapitata) | 385/464 | 264/327 | 74/93 | 47/44 |
Aquatic toxicity (Crustaceans) | 326/369 | 236/279 | 53/54 | 37/36 |
Aquatic toxicity (D. magna) | 302/287 | 221/216 | 45/41 | 36/30 |
Aquatic toxicity (Fish) | 597/831 | 436/628 | 102/125 | 59/78 |
Aquatic toxicity (Fathead minnow) | 437/424 | 312/322 | 79/56 | 46/46 |
Aquatic toxicity (Bluegill sunfish) | 264/504 | 195/382 | 42/77 | 27/45 |
Aquatic toxicity (Rainbow trout) | 217/553 | 156/422 | 41/81 | 20/50 |
Aquatic toxicity (Sheepshead minnow) | 102/228 | 84/168 | 11/38 | 7/22 |
Aquatic toxicity (T. pyriformis, classify) | 337/965 | 250/735 | 51/150 | 36/80 |
Aquatic toxicity (T. pyriformis) | 1310 | 991 | 202 | 117 |
BCF classify | 488/156 | 346/107 | 91/30 | 51/19 |
BCF | 463 | 331 | 79 | 53 |
Biodegradability | 906/550 | 644/401 | 158/94 | 104/55 |
Photoinduced toxicity | 659/627 | 473/458 | 118/115 | 68/54 |
Phototoxicity/Photoirritation | 221/146 | 150/108 | 43/26 | 28/12 |
Photoallergy | 660/462 | 475/333 | 117/85 | 68/44 |
Properities | Test set | Validation set | ||||||
---|---|---|---|---|---|---|---|---|
PCC | R2 | MAE | RMSE | PCC | R2 | MAE | RMSE | |
logS | 0.961 | 0.924 | 0.459 | 0.603 | 0.956 | 0.915 | 0.499 | 0.69 |
logP | 0.968 | 0.936 | 0.346 | 0.495 | 0.967 | 0.935 | 0.363 | 0.507 |
pKa | 0.803 | 0.645 | 1.054 | 2.014 | 0.869 | 0.755 | 0.924 | 1.63 |
Acidic pKa | 0.883 | 0.78 | 0.918 | 1.626 | 0.903 | 0.815 | 0.829 | 1.449 |
Basic pKa | 0.781 | 0.61 | 1.046 | 2.183 | 0.893 | 0.797 | 0.85 | 1.565 |
Caco-2 | 0.857 | 0.735 | 0.306 | 0.402 | 0.818 | 0.669 | 0.33 | 0.419 |
PPB | 0.814 | 0.662 | 0.108 | 0.154 | 0.846 | 0.716 | 0.107 | 0.151 |
VDss | 0.778 | 0.605 | 0.322 | 0.447 | 0.819 | 0.671 | 0.309 | 0.422 |
T1/2 | 0.506 | 0.256 | 0.43 | 0.593 | 0.599 | 0.358 | 0.379 | 0.544 |
MRT | 0.523 | 0.273 | 0.449 | 0.615 | 0.603 | 0.364 | 0.383 | 0.542 |
Neurotoxicity | 0.817 | 0.668 | 0.238 | 0.312 | 0.752 | 0.566 | 0.199 | 0.293 |
Mouse carcinogenicity | 0.68 | 0.462 | 0.729 | 0.922 | 0.72 | 0.518 | 0.851 | 1.085 |
Rat carcinogenicity | 0.69 | 0.476 | 0.864 | 1.071 | 0.61 | 0.372 | 1.095 | 1.334 |
Acute oral toxicity | 0.77 | 0.592 | 0.378 | 0.534 | 0.765 | 0.585 | 0.387 | 0.54 |
FDAMDD | 0.585 | 0.342 | 0.738 | 1.025 | 0.755 | 0.57 | 0.525 | 0.693 |
Aquatic toxicity (T. pyriformis) | 0.937 | 0.879 | 0.283 | 0.39 | 0.945 | 0.892 | 0.274 | 0.35 |
BCF | 0.858 | 0.737 | 0.505 | 0.645 | 0.853 | 0.727 | 0.511 | 0.684 |
Properities | Test set | Validation set | ||||||
---|---|---|---|---|---|---|---|---|
AUC | Precision | Recall | Specificity | AUC | Precision | Recall | Specificity | |
Caco-2 classify | 0.95 | 0.846 | 0.898 | 0.878 | 0.905 | 0.795 | 0.816 | 0.84 |
HIA | 0.977 | 0.961 | 0.936 | 0.824 | 1.0 | 0.978 | 1.0 | 0.5 |
MDCK | 0.838 | 0.862 | 0.641 | 0.879 | 0.786 | 0.773 | 0.739 | 0.783 |
F50% | 0.78 | 0.738 | 0.756 | 0.654 | 0.808 | 0.771 | 0.73 | 0.68 |
F30% | 0.792 | 0.802 | 0.892 | 0.514 | 0.868 | 0.881 | 0.875 | 0.68 |
F20% | 0.763 | 0.826 | 0.937 | 0.354 | 0.879 | 0.92 | 0.908 | 0.647 |
BBB | 0.927 | 0.932 | 0.983 | 0.69 | 0.946 | 0.917 | 0.974 | 0.685 |
OATP1B1 inhibitor | 0.823 | 0.939 | 0.943 | 0.462 | 0.709 | 0.967 | 0.98 | 0.444 |
OATP1B3 inhibitor | 0.891 | 0.947 | 0.983 | 0.235 | 0.889 | 0.963 | 1.0 | 0.143 |
OATP2B1 inhibitor | 0.851 | 0.333 | 0.143 | 0.909 | 1.0 | 0.0 | 0.0 | 1.0 |
OCT1 inhibitor | 0.889 | 0.6 | 0.6 | 0.889 | 1.0 | 1.0 | 0.5 | 1.0 |
OCT2 inhibitor | 0.84 | 0.643 | 0.29 | 0.94 | 0.839 | 0.625 | 0.357 | 0.946 |
BCRP inhibitor | 0.886 | 0.794 | 0.89 | 0.761 | 0.861 | 0.724 | 0.913 | 0.709 |
BSEP inhibitor | 0.883 | 0.776 | 0.865 | 0.675 | 0.938 | 0.838 | 0.939 | 0.714 |
MATE1 inhibitor | 0.851 | 0.0 | 0.0 | 1.0 | 0.733 | 0.0 | 0.0 | 1.0 |
Pgp inhibitor | 0.924 | 0.837 | 0.938 | 0.678 | 0.892 | 0.836 | 0.939 | 0.714 |
Pgp substrate | 0.839 | 0.75 | 0.745 | 0.765 | 0.826 | 0.721 | 0.778 | 0.759 |
CYP1A2 inhibitor | 0.912 | 0.809 | 0.837 | 0.826 | 0.922 | 0.824 | 0.873 | 0.834 |
CYP3A4 inhibitor | 0.901 | 0.701 | 0.735 | 0.871 | 0.897 | 0.74 | 0.715 | 0.885 |
CYP2B6 inhibitor | 0.741 | 0.571 | 0.48 | 0.83 | 0.817 | 0.667 | 0.737 | 0.788 |
CYP2C9 inhibitor | 0.888 | 0.732 | 0.759 | 0.852 | 0.895 | 0.781 | 0.768 | 0.87 |
CYP2C19 inhibitor | 0.897 | 0.796 | 0.836 | 0.827 | 0.909 | 0.79 | 0.845 | 0.813 |
CYP2D6 inhibitor | 0.847 | 0.67 | 0.524 | 0.935 | 0.872 | 0.73 | 0.548 | 0.942 |
CYP1A2 substrate | 0.923 | 0.758 | 0.758 | 0.878 | 0.914 | 0.68 | 0.756 | 0.856 |
CYP3A4 substrate | 0.873 | 0.78 | 0.786 | 0.802 | 0.867 | 0.721 | 0.855 | 0.767 |
CYP2B6 substrate | 0.885 | 0.78 | 0.852 | 0.735 | 0.856 | 0.722 | 0.765 | 0.8 |
CYP2C9 substrate | 0.857 | 0.58 | 0.554 | 0.861 | 0.851 | 0.667 | 0.566 | 0.896 |
CYP2C19 substrate | 0.944 | 0.816 | 0.827 | 0.922 | 0.912 | 0.727 | 0.632 | 0.905 |
CYP2D6 substrate | 0.885 | 0.726 | 0.625 | 0.886 | 0.92 | 0.79 | 0.754 | 0.904 |
HLM | 0.85 | 0.743 | 0.661 | 0.882 | 0.822 | 0.669 | 0.552 | 0.861 |
RLM | 0.813 | 0.763 | 0.829 | 0.624 | 0.828 | 0.772 | 0.853 | 0.647 |
UGT substrate | 0.806 | 0.768 | 0.729 | 0.757 | 0.716 | 0.676 | 0.754 | 0.577 |
CLp | 0.807 | 0.713 | 0.72 | 0.722 | 0.792 | 0.675 | 0.628 | 0.79 |
CLr | 0.685 | 0.592 | 0.913 | 0.341 | 0.517 | 0.641 | 0.833 | 0.222 |
DILI | 0.716 | 0.742 | 0.715 | 0.615 | 0.707 | 0.7 | 0.74 | 0.536 |
hERG 1uM | 0.868 | 0.654 | 0.614 | 0.92 | 0.879 | 0.561 | 0.587 | 0.916 |
hERG 10uM | 0.884 | 0.803 | 0.894 | 0.675 | 0.88 | 0.761 | 0.912 | 0.641 |
hERG 30uM | 0.919 | 0.906 | 0.963 | 0.637 | 0.908 | 0.854 | 0.968 | 0.485 |
hERG 1-10uM | 0.946 | 0.806 | 0.873 | 0.896 | 0.96 | 0.679 | 0.905 | 0.851 |
hERG 10-30uM | 0.941 | 0.92 | 0.958 | 0.769 | 0.938 | 0.869 | 0.96 | 0.667 |
Respiratory toxicity | 0.913 | 0.889 | 0.804 | 0.866 | 0.904 | 0.827 | 0.796 | 0.839 |
Nephrotoxicity | 0.777 | 0.719 | 0.695 | 0.789 | 0.744 | 0.655 | 0.613 | 0.615 |
Eye corrosion | 0.985 | 0.886 | 0.986 | 0.92 | 0.98 | 0.923 | 0.95 | 0.937 |
Eye irritation | 0.951 | 0.921 | 0.981 | 0.736 | 0.978 | 0.944 | 0.991 | 0.828 |
Skin corrosion | 0.931 | 0.785 | 0.848 | 0.866 | 0.937 | 0.865 | 0.828 | 0.91 |
Skin irritation | 0.782 | 0.698 | 0.77 | 0.673 | 0.794 | 0.683 | 0.76 | 0.672 |
Skin sensitisation | 0.915 | 0.8 | 0.727 | 0.881 | 0.854 | 0.683 | 0.737 | 0.809 |
Acute dermal toxicity | 0.848 | 0.835 | 0.722 | 0.812 | 0.764 | 0.689 | 0.662 | 0.685 |
Ames mutagenesis | 0.88 | 0.808 | 0.837 | 0.745 | 0.855 | 0.773 | 0.833 | 0.726 |
Mouse carcinogenicity classify | 0.718 | 0.644 | 0.613 | 0.687 | 0.704 | 0.618 | 0.636 | 0.698 |
Rat carcinogenicity classify | 0.708 | 0.615 | 0.709 | 0.598 | 0.779 | 0.75 | 0.78 | 0.705 |
Rodents carcinogenicity | 0.731 | 0.64 | 0.679 | 0.557 | 0.85 | 0.776 | 0.809 | 0.738 |
Micronucleus | 0.949 | 0.767 | 0.868 | 0.873 | 0.936 | 0.85 | 0.85 | 0.923 |
Reproductive toxicity | 0.887 | 0.939 | 0.655 | 0.963 | 0.885 | 0.9 | 0.659 | 0.933 |
Mitochondrial toxicity | 0.908 | 0.843 | 0.836 | 0.853 | 0.914 | 0.829 | 0.817 | 0.853 |
Hemolytic toxicity | 0.847 | 0.709 | 0.847 | 0.709 | 0.845 | 0.721 | 0.738 | 0.765 |
Repeated dose toxicity | 0.732 | 0.741 | 0.827 | 0.544 | 0.736 | 0.761 | 0.875 | 0.492 |
Acute oral toxicity classify | 0.892 | 0.892 | 0.859 | 0.777 | 0.87 | 0.828 | 0.867 | 0.721 |
FDAMDD classify | 0.829 | 0.76 | 0.76 | 0.791 | 0.894 | 0.783 | 0.878 | 0.744 |
AR | 0.851 | 0.649 | 0.363 | 0.981 | 0.934 | 0.526 | 0.435 | 0.969 |
ER | 0.902 | 0.72 | 0.462 | 0.987 | 0.949 | 0.586 | 0.531 | 0.981 |
AR-LBD | 0.863 | 0.574 | 0.39 | 0.972 | 0.931 | 0.591 | 0.5 | 0.969 |
ER-LBD | 0.867 | 0.546 | 0.44 | 0.952 | 0.934 | 0.558 | 0.509 | 0.959 |
Aromatase | 0.822 | 0.565 | 0.416 | 0.955 | 0.85 | 0.481 | 0.4 | 0.944 |
AhR | 0.864 | 0.557 | 0.412 | 0.955 | 0.869 | 0.617 | 0.483 | 0.964 |
ARE | 0.856 | 0.604 | 0.384 | 0.946 | 0.884 | 0.655 | 0.603 | 0.954 |
ATAD5 | 0.835 | 0.765 | 0.191 | 0.996 | 0.917 | 0.6 | 0.222 | 0.993 |
HSE | 0.852 | 0.625 | 0.185 | 0.994 | 0.831 | 0.5 | 0.172 | 0.991 |
p53 | 0.866 | 0.455 | 0.205 | 0.983 | 0.884 | 0.5 | 0.242 | 0.986 |
PPARγ | 0.922 | 0.274 | 0.586 | 0.939 | 0.296 | 0.615 | ||
MMP | 0.93 | 0.755 | 0.669 | 0.955 | 0.949 | 0.75 | 0.789 | 0.952 |
TR | 0.865 | 0.636 | 0.233 | 0.99 | 0.857 | 0.571 | 0.133 | 0.993 |
GR | 0.906 | 0.812 | 0.544 | 0.985 | 0.912 | 0.812 | 0.531 | 0.988 |
Honey bee toxicity | 0.799 | 0.579 | 0.367 | 0.907 | 0.955 | 0.923 | 0.667 | 0.978 |
Avain toxicity (Colinus virginanus) | 0.764 | 0.636 | 0.368 | 0.953 | 1.0 | 1.0 | 1.0 | 1.0 |
Avain toxicity (Anas platyrhynchos) | 0.821 | 0.727 | 0.421 | 0.966 | 0.907 | 0.8 | 0.667 | 0.976 |
Aquatic toxicity (P. subcapitata) | 0.895 | 0.821 | 0.897 | 0.729 | 0.811 | 0.667 | 0.864 | 0.596 |
Aquatic toxicity (Crustaceans) | 0.888 | 0.768 | 0.828 | 0.765 | 0.872 | 0.829 | 0.806 | 0.838 |
Aquatic toxicity (D. magna) | 0.895 | 0.741 | 0.833 | 0.788 | 0.856 | 0.815 | 0.733 | 0.861 |
Aquatic toxicity (Fish) | 0.911 | 0.826 | 0.902 | 0.721 | 0.91 | 0.829 | 0.872 | 0.763 |
Aquatic toxicity (Fathead minnow) | 0.911 | 0.843 | 0.843 | 0.817 | 0.891 | 0.844 | 0.826 | 0.848 |
Aquatic toxicity (Bluegill sunfish) | 0.92 | 0.848 | 0.918 | 0.709 | 0.942 | 0.878 | 0.956 | 0.778 |
Aquatic toxicity (Rainbow trout) | 0.893 | 0.852 | 0.945 | 0.647 | 0.916 | 0.9 | 0.9 | 0.75 |
Aquatic toxicity (Sheepshead minnow) | 0.938 | 0.818 | 0.957 | 0.643 | 0.89 | 0.955 | 0.955 | 0.857 |
Aquatic toxicity (T. pyriformis, classify) | 0.974 | 0.931 | 0.964 | 0.821 | 0.949 | 0.923 | 0.9 | 0.833 |
BCF classify | 0.958 | 0.583 | 0.875 | 0.851 | 0.943 | 0.739 | 0.895 | 0.882 |
Biodegradability | 0.839 | 0.628 | 0.772 | 0.753 | 0.872 | 0.643 | 0.818 | 0.76 |
Photoinduced toxicity | 0.712 | 0.659 | 0.68 | 0.656 | 0.743 | 0.633 | 0.704 | 0.676 |
Phototoxicity/Photoirritation | 0.719 | 0.609 | 0.467 | 0.8 | 0.747 | 0.438 | 0.583 | 0.679 |
Photoallergy | 0.703 | 0.6 | 0.6 | 0.73 | 0.744 | 0.667 | 0.636 | 0.794 |
admetSAR3.0 was designed with HTML, CSS, and JavaScript for the frontend, utilizing jQuery for page control and event handling. It incorporates JSME as a molecular editor and employs ApacheECharts for data visualization. The core logic of the website is implemented in PHP, with mariaDB chosen as the data management system. The prediction models were built with Python, and the modeling process was supported by chemical informatics packages such as PyTorch, DGL, DGLlife, and RDKit. The server has been successfully tested on Google Chrome, Microsoft Edge, Mozilla Firefox, and Apple Safari.
Software and tool | Version |
---|---|
PHP | 5.4.16 |
MySQL | 5.5.64 |
Anaconda | 4.12.0 |
NVIDIA CUDA Toolkit | 11.5 |
Python | 3.9.18 |
RDKit | 2022.03.2 |
dgl | 0.9.1 |
dgllife | 0.3.2 |
pytorch | 1.11.0 |
torchvision | 0.12.0 |
jQuery | 2.1.1 |
Bootstrap | 3.3.7 |
JSME | 2022-06-10 |
Apache ECharts | 5.4.0 |
OS | Version | Chrome | Edge | Firefox | Safari |
---|---|---|---|---|---|
Linux | Ubuntu 18.04.6 LTS | 79.0.3945.88 | - | 74.0.1 | - |
MacOS | Catalina 10.15.7 | 75.0.3770.80 | - | 71.0 | 13.1 |
Windows | 10 | 75.0.3770.80 | 80.0.361.109 | 71.0 | - |