Laboratory of Molecular Modeling and Design
Introduction
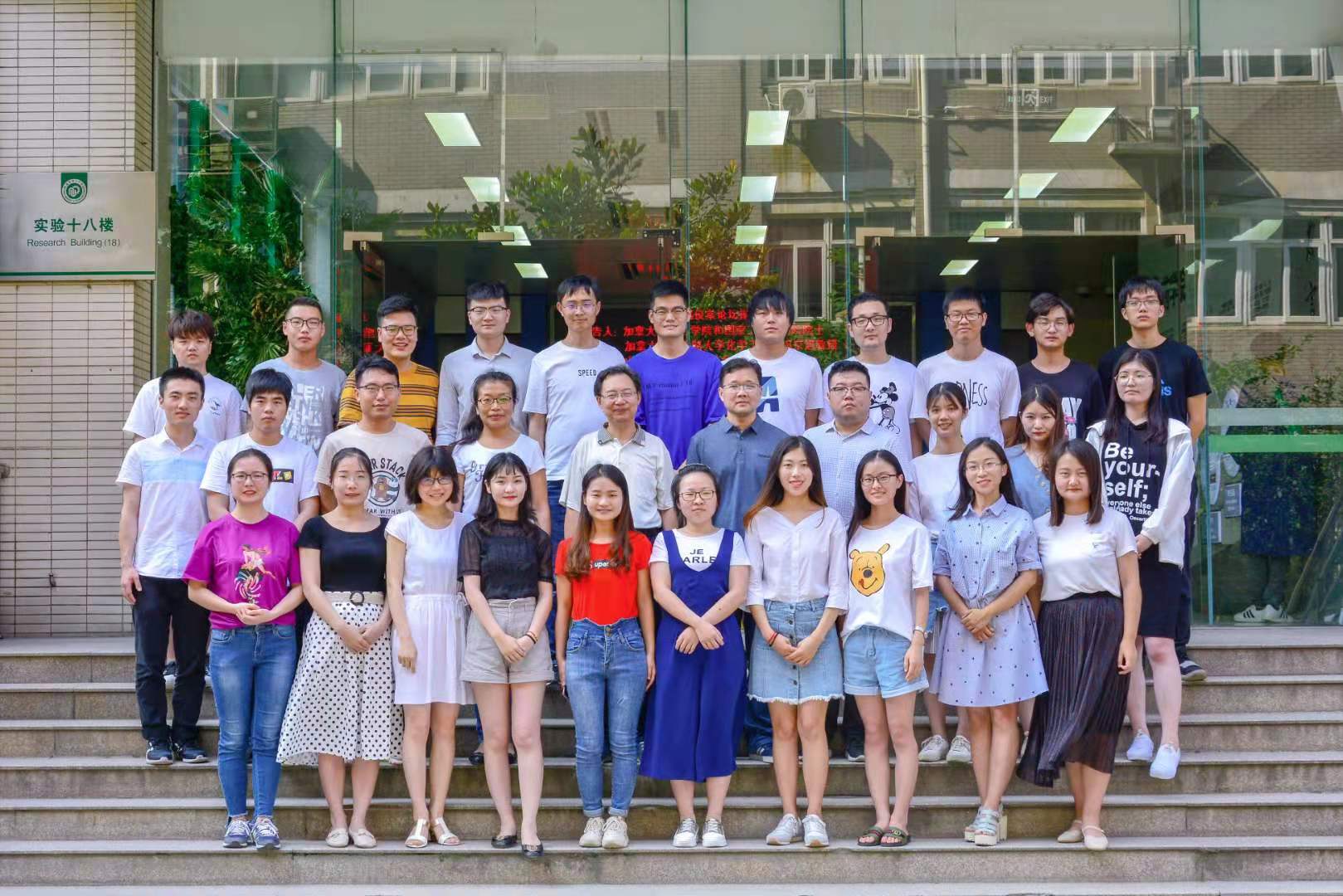
Laboratory of Molecular Modeling and Design was established in September 2004, the first lab in School of Pharmacy. Now we have built a computer-aided drug design research platform and a scientific research team. The laboratory has equipped with powerful computer facilities and molecular modeling and design software.
At present, there are 34 members in the laboratory, including 3 professors, 1 PostDoc, 11 Ph.D. students and 19 Master students. Furthermore, several undergraduate students also participate in our research. (updated in May 2021)
Main Research Directions
Our researches mainly focus on computational simulations of biological complex systems, computer-aided drug design, algorithms and databases development, etc. By collaborating with many other groups, we contribute in discovering and design drugs on anti-viral, anti-tumor, nervous system disease and metabolism related diseases. We also work on developing drug design methods, such as database construction, pharmacokinetic properties and toxicity (ADMET) prediction and multi-target drug design. Until now, we have published a lot of papers cited by SCI including PLoS Comput. Biol., J. Med. Chem., J. Chem. Theory Comput., J. Phys. Chem. B, J. Chem. Inf. Model., Proteins, PLoS One., Angew. Chem. Int. Ed., etc. Five Chinese patents and two software copyrights were also applied.
Research Projects
Estrogen receptors-based Drugs Design
Estrogen receptors (ER) belong to the nuclear receptor superfamily, and two subtypes, ERα and ERβ, have been identified to date.
The differentiated functions and receptor expressions of ERα and ERβ made it attracted to discover subtype-specified ligands with high selectivity.
By molecular dynamics and steered molecular dynamics simulations, the mechanism of ligand selectivity of ER subtypes were investigated by us
(J. Phys. Chem. B, 2008, 112: 2719–2726, J. Phys. Chem. B 2009, 113: 10436–10444).
With virtual screening based on a structure optimized through molecular dynamics (MD) and bioassays,
18 potent ligands of estrogen receptor (ER) β were discovered from 70 purchased compounds here (J. Med. Chem. 2010, 53, 5361–5365).
These findings could be helpful for rational design of highly selective ER ligands for breast cancer treatment.
Computational Simulations of Biological Complex Systems
CYP450-based Computational Simulations. The human cytochromes P450 (CYPs), a superfamily of heme-containing enzymes with about 57 isoforms, catalyze the metabolism of a variety of endogenous and xenobiotic compounds. CYP2A6 is the major enzyme to catalyze coumarin 7-hydroxylation, and this enzyme also plays an important role in the metabolism of nicotine and other tobacco-specific compounds. Recent experimental data showed that the N297S and A481T mutants of CYP2A6 decreased the catalytic activity toward coumarin by about 4-fold and 10-fold, respectively. These two mutants also had about 30-fold decrease in binding affinity for coumarin when compared to its wild type. At present, however, how the mutations affect the enzymatic activity and/or the substrate binding remains unclear. In this study, a combination of molecular docking and molecular dynamics (MD) simulation was employed to investigate the above question. Our results demonstrated that the N297S mutation altered the hydrogen-bonding network mediated by a water molecule between the B'−C loop and the I helix and thus a shift of the B'helix/B'−C loop region, whereas the A481T mutation triggered the conformational changes of its adjacent residues including Phe209 and Phe280 via an indirect manner to affect the substrate binding (J. Chem. Theory Comput., 2009, 5: 1411–1420). Recently, we used the random acceleration molecular dynamics simulations combined with steered molecular dynamics and potential of mean force calculations to investigate the possible unbinding pathways in CYP2E1. Two most likely unbinding channels of CYP2E1 were identified by our study (PLoS ONE, 2012, 7: e33500).
In Silico ADMET Properties Prediction
Database and Algorithms Development. There are numerous small molecular compounds around us to affect our health, such as drugs,
pesticides, food additives, industrial chemicals, and environmental pollutants. Over decades, properties related to absorption, distribution, metabolism,
excretion, and toxicity (ADMET) have become one of the most important issues to assess the effects or risks of these compounds in human body.
Since in vivo and in vitro evaluations are costly and laborious, in silico techniques have been widely used to estimate these properties.
In the past four years, several novel methods, such as substructure pattern recognition (J. Chem. Inf. Model. 2010, 50: 1034-041.),
combined classifiers (J. Chem. Inf. Model. 2011, 51: 996-1011). etc were developed by us. This method has been used to study currently available data sets,
including human intenstinal absorption, BBB penetration, CYP450 inhibition, environmental toxicities and biodegradability. Recently,
an ADMET structure-activity relationship server, abbreviated as admetSAR was developed by us. The admetSAR is a comprehensive source
and free tool for in silico evaluating ADMET properties. In admetSAR, over 210,000 ADMET annotated data points for more than 96,000 unique compounds with
45 ADMET-associated proteins, species or organisms have been manually curated from large number of literatures. The server provides a user-friendly
interface to search a specific chemical profile, by CASRN, common name or similarity. In addition, more than 20 predictive QSAR models were implemented
to predict ecological/mammalian ADMET potential of new chemicals.
Drug-Target Interaction Prediction and Drug Repositioning
Study of drug-target interaction (DTI) networks is an important topic toward the elucidation of protein functions and the understanding of the molecular
mechanisms inside the cell. It is both time consuming and costly to determine potential DTI by experiments alone. Computational methods for the large-scale
analysis and prediction of DTI and identification of drugs having polypharmacology profiles complement research in chemical genetics and chemogenomics.
Recently, we developed three supervised inference methods, namely drug-based similarity inference, target-based similarity inference and network-based inference
(NBI) to predict new DTI and drug repositioning. Via NBI some new DTI were predicted based on 12,483 FDA approved and experimental drug-target links.
In vitro assays confirmed that five old drugs showed novel polypharmacological features on estrogen receptors or dipeptidyl peptidase-IV with IC50 or EC50
values ranged from 200nM to 10uM. And two drugs also showed potent antiproliferative activities on human MDA-MB-231 breast cancer cell line in MTT assays.
In addition, we also developed a computational tool based on multitarget-Quantitative Structure-Activity Relationship (mt-QSAR) and computational
chemogenomics methods, namely CPI-Predictor: http://www.lmmd.org/software.html, for compound-protein interactions (CPI) prediction.
Our methods could be powerful tools in prediction of DTI and drug repositioning.
Quick link to our publications
![]() |
![]() |
![]() |
![]() |
![]() |
![]() |
![]() |